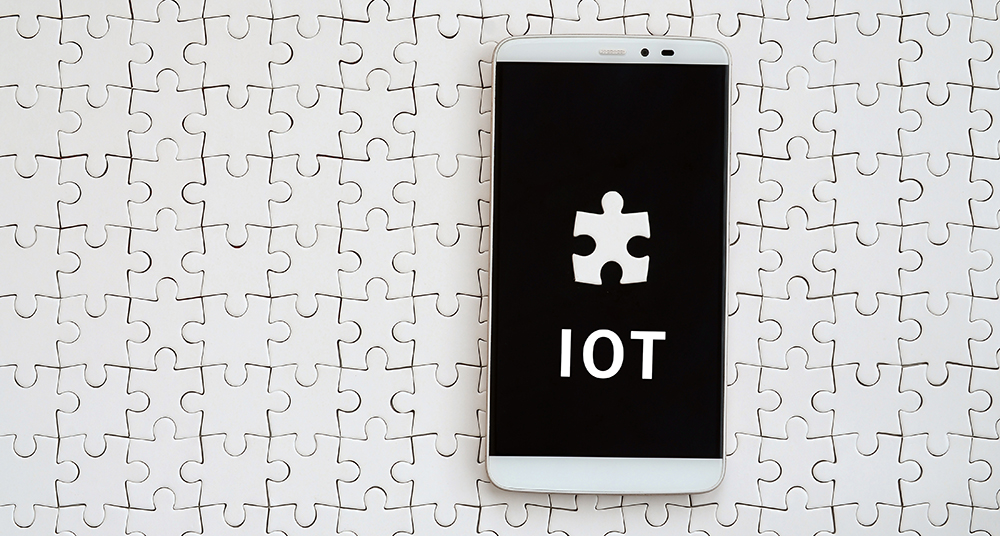
After years of being on the cusp of broad adoption, IoT still hasn’t reached a fraction of its potential. The majority of executives in the 2019 PwC IoT survey said their business has one or more IoT projects in progress, but many admit that several issues have slowed or thwarted their progress.
It’s relatively easy to put together an IoT proof of concept (PoC) that sends device data to the cloud, but the challenges get more complex when deploying enterprise-grade IoT at scale. These challenges include integration with factory systems managed by OT (operations technology) and enterprise systems, security of data and critical infrastructure, gaining insights from device data, and doing all of this without breaking the bank.
The environment for enterprise IoT dictates far more complex requirements than what it takes to build a PoC or put together a consumer IoT solution that uses fully integrated, but proprietary solutions. The PoCs using consumer components are not going to be integrated with the company’s existing IT infrastructure and will operate in their own silo, requiring them to have their own database, network, security, apps and analytics. While quick to assemble, they’re designed for one-time use and lack the rigor of production quality testing, security policies, maintenance planning and ongoing support.
Additionally, these PoCs typically have a 2-tier architecture where data acquisition is at the edge but where processing/analytics and intelligence are in the cloud. The cloud service providers make it easy to connect to their services by providing pre-configured runtimes. However, factors like cost, data privacy and network latencies make it unviable to scale deployments beyond the PoC. As a result, most PoCs fail to scale and make any real difference beyond the business group that sponsored them.
Using edge computing to scale IoT
The real value of IoT lies in gaining insights by integrating device data with data from enterprise, customers and supply chain. However, before the device data is integrated, it needs to be transformed from one protocol to another, cleaned and routed to applications that need to act on it.
In some scenarios, the device data needs to be acted upon in real time with business-critical functions continuing even with loss of network connectivity, a must for life-preserving safety systems or critical infrastructure.
Edge computing can solve these IoT challenges by bringing data processing and analytics closer to devices where data is being generated. The device data can be prioritized and routed to when and where it's needed (e.g. critical alerts can be prioritized and routed to a different endpoint.) Instead of all the device data, only a small subset of data needs to be sent to the core site for long term storage or further processing.
Artificial intelligence and machine learning (AI/ML) allows companies to proactively discover potential issues in real time so corrective action can be taken to improve product quality, reducing potential downtime through predictive maintenance. This requires data scientists to develop, test and deploy ML models for predictive maintenance. These models then are deployed for real time inference at the edge sites.
Unlike the embedded systems of the past that were custom built and managed by operations technology, the desired approach is to not only collect, analyze and act on device data in real time, but to do so in a standardized manner.
To achieve this vision, companies must now take a hard look at their operations and technology. It requires the IoT solution to mirror best practices of a modern IT environment based on containers, Kubernetes, agile development, AI/ML and automation. This approach will allow companies to quickly adapt to changes and not be overwhelmed by faster moving competitors.
The enterprise considerations not only involve building an IoT solution, but also how to deploy this solution to thousands of sites, day-two operations, and managing infrastructure and application lifecycle at various locations with existing IT resources.
Consistent approach covering multiple use cases
Using a modular approach to building the edge architecture provides the flexibility to deploy any of the components shown where it makes sense - from the core data center to the remote edge sites, to address the needs of various use cases including IoT.
By using open APIs, developers can access data in the programmatic manner, e.g. front-end developers can access IoT device data without worrying about the hardware interfaces or device drivers. Similarly, an IoT with open source components will allow companies to build vendor agnostic solutions that best fits their needs from core to edge.
About the author: Ishu Verma is Technical Evangelist at Red Hat focused on emerging technologies like edge computing and IoT. He and fellow open source hackers work on building solutions with next gen open source technologies. Previously at Intel, Ishu helped bring IoT Gateways to market and build end-to-end IoT solutions with partners.
Edited by
Ken Briodagh